About me
I am a researcher at StepFun, working with Dr. Gang Yu on advancing AI-generated content (AIGC), personalized content creation, 3D generation, neural rendering, and computer graphics. I am focused on applying advanced AI techniques within the field of creative content to explore its potential applications. Prior to joining StepFun, I held research roles at Tencent, SenseTime Research, and Shanghai AI Lab.
Selected Projects
-
MVPaint
MVPaint explores synchronized multi-view diffusion to create consistent and detailed 3D textures from textual descriptions. With synchronized multi-view diffusion, it delivers seamless, high-res textures with minimal UV wrapping dependency.
>> Project Page -
MeshXL
MeshXL is a family of generative pre-trained foundation models for 3D mesh generation. With the Neural Coordinate Field representation, the generation of unstructured 3D mesh data can be seaminglessly addressed by modern LLM methods.
>> Project Page -
DNA-Rendering
DNA-Rendering is a large-scale, high-fidelity repository of human performance data for neural actor rendering, which contains large volume of data with diverse attributes and rich annotation. Along with the dataset, a large-scale and quantitative benchmark in full-scale with multiple tasks on human rendering is provided.
>> Project Page -
GNR & GeneBody
Generalizable Neural Performer (GNR) learns a generalizable and robust neural body representation over various geometry and appearance with a Geometric Body Embedding strategy which achors body shape priors to implicit field and Screen-Space Occlusion-Aware Appearance Blending to help image blending from source views. A dataset GeneBody is constructed to demonstrate the effectness of the proposed algorithm.
>> Project Page -
Renderme-360
RenderMe-360 is a comprehensive 4D human head dataset to drive advance in head avatar research which contains massive data assets with high-fidelity, high deversity and rich annotation attributions. A comprehensive benchmark for head avatar research, with 16 state-of-the-art methods performed on five main tasks which opens the door for future exploration in head avatars.
>> Project Page -
MonoHuman
MonoHuman, which robustly renders view-consistent and high-fidelity avatars under arbitrary novel poses from monocular videos. The key insight is to model the deformation field with bi-directional constraints and explicitly leverage the off-the-peg keyframe information to reason the feature correlations for coherent results. Extensive experiments demonstrate the superiority of our proposed MonoHuman over state-of-the-art methods.
>> Project Page
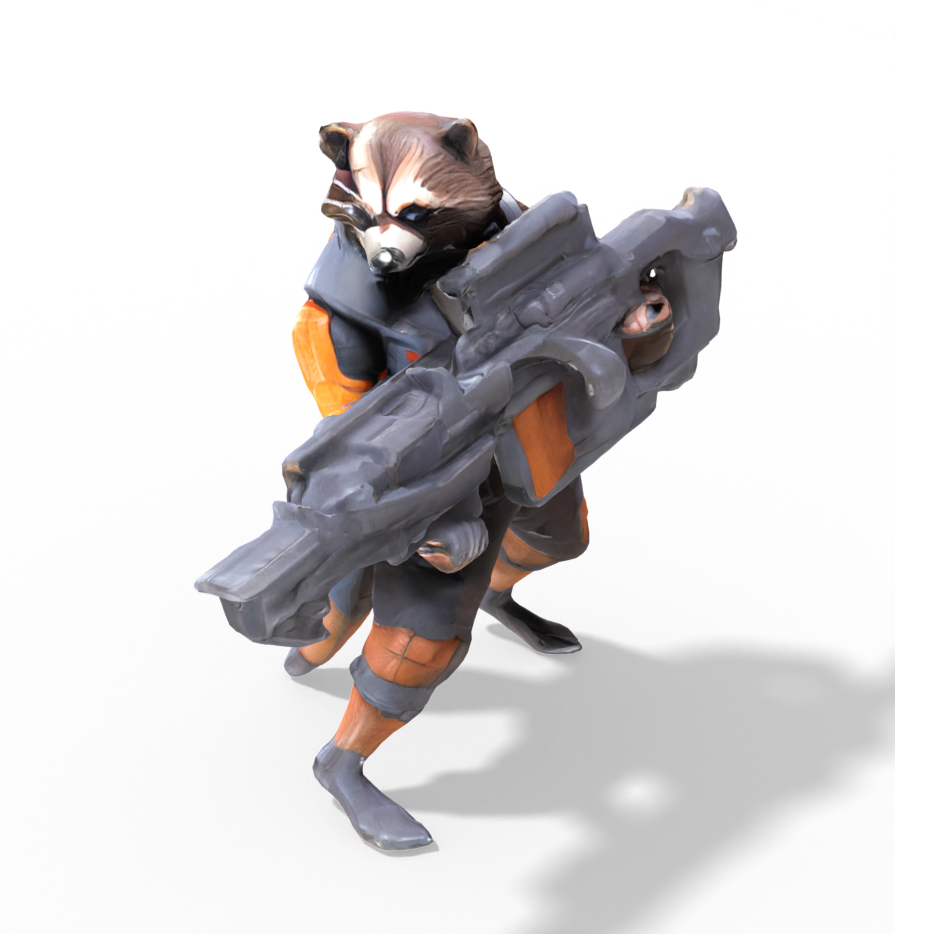